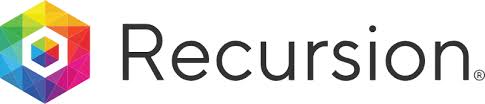
Recapitulating known compound-gene relationships
This benchmark evaluates the zero-shot prediction of compound-gene activity.
Performance is measured separately for each compound using Average Precision and AUC-ROC. Per-compound measures are then aggregated into one global performance metric.
Maps of Biology and Chemistry
At Recursion, we build maps of biology and chemistry to explore uncharted areas of disease biology, unravel its complexity, and industrialize drug discovery. We use deep learning models to embed high dimensional representations of biology (e.g. phenomics, transcriptomics). This allows us to create representations that can be compared and contrasted to predict trillions of relationships across biology and chemistry — even without physically testing all of the possible combinations. Just as a map helps to navigate the physical world, our maps are designed to help us understand as much as we can about the connectedness of human biology so we can navigate the path to new medicines more efficiently.
We evaluated our recently released OpenPhenom-S/16
model, as well as our proprietary Phenom-1
and Phenom-2
models. Can you do better?
.png)
Resources
This benchmark was released alongside RxRx3-Core
and OpenPhenom-S/16
.
RxRx3-Core
A challenge dataset in phenomics optimized for the research community. RxRx3-core includes labeled images of 735 genetic knockouts and 1,674 small-molecule perturbations drawn from the RxRx3 dataset, image embeddings computed with OpenPhenom-S/16, and associations between the included small molecules and genes. The dataset contains 6-channel Cell Painting images and associated embeddings from 222,601 wells but is less than 18Gb, making it incredibly accessible to the research community.
OpenPhenom-S/16
OpenPhenom-S/16is a foundation model that flexibly processes microscopy images into general-purpose embeddings. In other words, OpenPhenom-S/16 can take a series of microscopy channels and create a meaningful vector representation of the input image. This enables robust comparison of images, and other data science techniques to decode any biology or chemistry within such images. The precomputed embeddings of OpenPhenom-S/16 are included in the RxRx3-core dataset!